5 Things to Know About Buying AI for Your Business
by Aware
Thinking about your 2024 budget? Here’s 5 things you need to know before making an AI purchase.
“Artificial intelligence” encompasses a wide range of AI and machine learning (ML) models that can add value to your business—or create needless complexity at great cost. Before making an AI purchase, it’s essential to consider what use case the technology can solve, if the product you’re buying is really the best fit for your company, and if the model will remain relevant and cost-effective into the future.
As an AI-native business, Aware understands the complexities of implementing enterprise-grade AI. In this post, our data scientists share 5 top tips for making the right AI purchase for your business.
1. Not all AI is created equal
Artificial intelligence and machine learning capabilities range from incredibly simple (“Is there are a person in this picture?”) to extremely complex (“Safely drive this car through rush hour traffic”). It’s important to consider potential use cases for AI within your business to avoid overspending on technology that has no practical application.
Every AI vendor should be able to explain what models they use, how they work, and why they’re the most appropriate AI for your use case. Often, it will be more cost-effective—and deliver better results—to purchase smaller, highly targeted models that solve very specific needs within your organization.
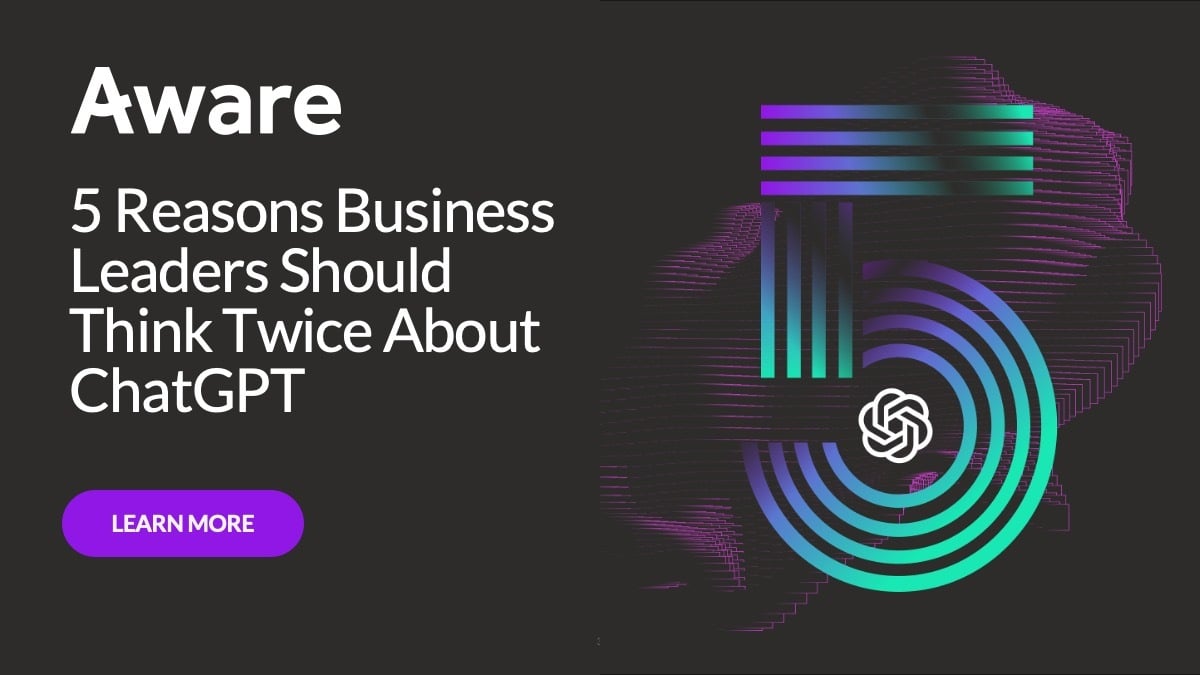
Opinion: Why AI needs a use case to be effective
2. AI models are unbelievably big
Generative AI large language models like those that power ChatGPT are trained on an extraordinary number of tokens, which are units of code or text such as words and characters. Relatively straightforward classification models might need around 20,000 high-quality tokens to train. LLMs like OpenAI’s GPT-4, Meta’s Llama-2, and Google’s Bard can contain tens of billions of parameters, and each one needs a minimum of 20-30 tokens to train.
That’s just the start of the problem of scale when it comes to AI. Models can demand significant resources as they grow, especially if they are expected to handle more data, users, and tasks without impacting performance. What starts as a cheap investment could quickly become prohibitively expensive as it scales, especially in enterprise settings. And even when cost isn’t a factor, the availability of computing resources needed to run the AI might still present problems for the business. For example, the global GPU shortage is anticipated to last through 2025, creating chaos for AI companies unable to scale without additional processing power.

Aware vs Llama-2—Who wins?
3. Garbage in = Garbage out
AI/ML models are only as good as the data used to train them. Because of the huge number of tokens training requires, and the cost and complexity of sourcing relevant data, it is always challenging for data scientists to access high enough quantities of good data at the right price.
Some solve this problem by ingesting as much free data as possible—OpenAI’s GPT-3 LLM used 45 terabytes of plain text to train its approximately 175 billion parameters. The vast majority of that data was content scraped from the internet, everything from blog posts and news articles to social media comments, fanfiction, and ebooks.
At that scale there’s no vetting the quality of the data, or even knowing everything the models ingested. While this method of training an AI/ML model can enhance performance in some areas, it can also lead to inaccuracies, “hallucinations,” and reduced performance in others.
An alternative methodology is to train models on closely curated datasets that have been carefully vetted to enhance output accuracy. Using train-validation-test datasets, model development can be further refined against benchmarks that make the results both knowable and explainable.
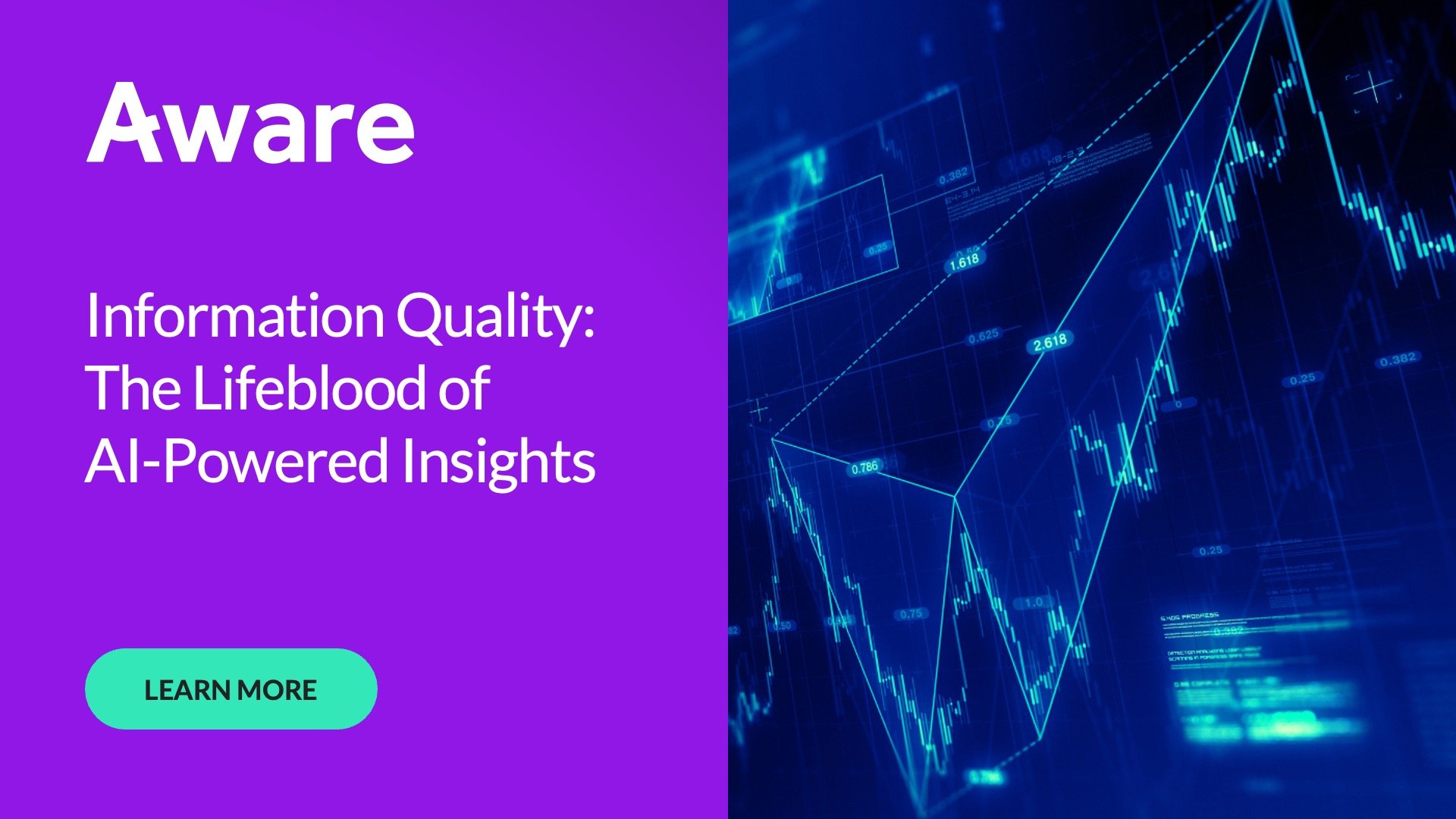
Introducing InfoQ: Aware's latest information quality model
4. AI models shouldn’t be static
The moment an AI model is released, it’s out of date. That doesn’t mean it’s automatically useless, but refreshing the models should be on the roadmap to keep the technology useful and relevant. Balancing the cost and complexity of updating the AI with performance degradation can look different for every model, with some needing to be refreshed on a regular basis while others can go a year or more without an update.
Continually assessing the model’s performance while in use is essential for understanding how accurate it is, and how well it responds to the demands placed on it in terms of resource consumption, API usage, and inference speed. It’s also important to remember that the data the model ingests is in a continuous state of flux. “Drift” refers to the changes to this data over time, and it has considerable impact on model output and reliability. For example, a natural language processing (NLP) model that isn’t regularly updated will struggle to keep up with changes in word meanings and emerging slang.
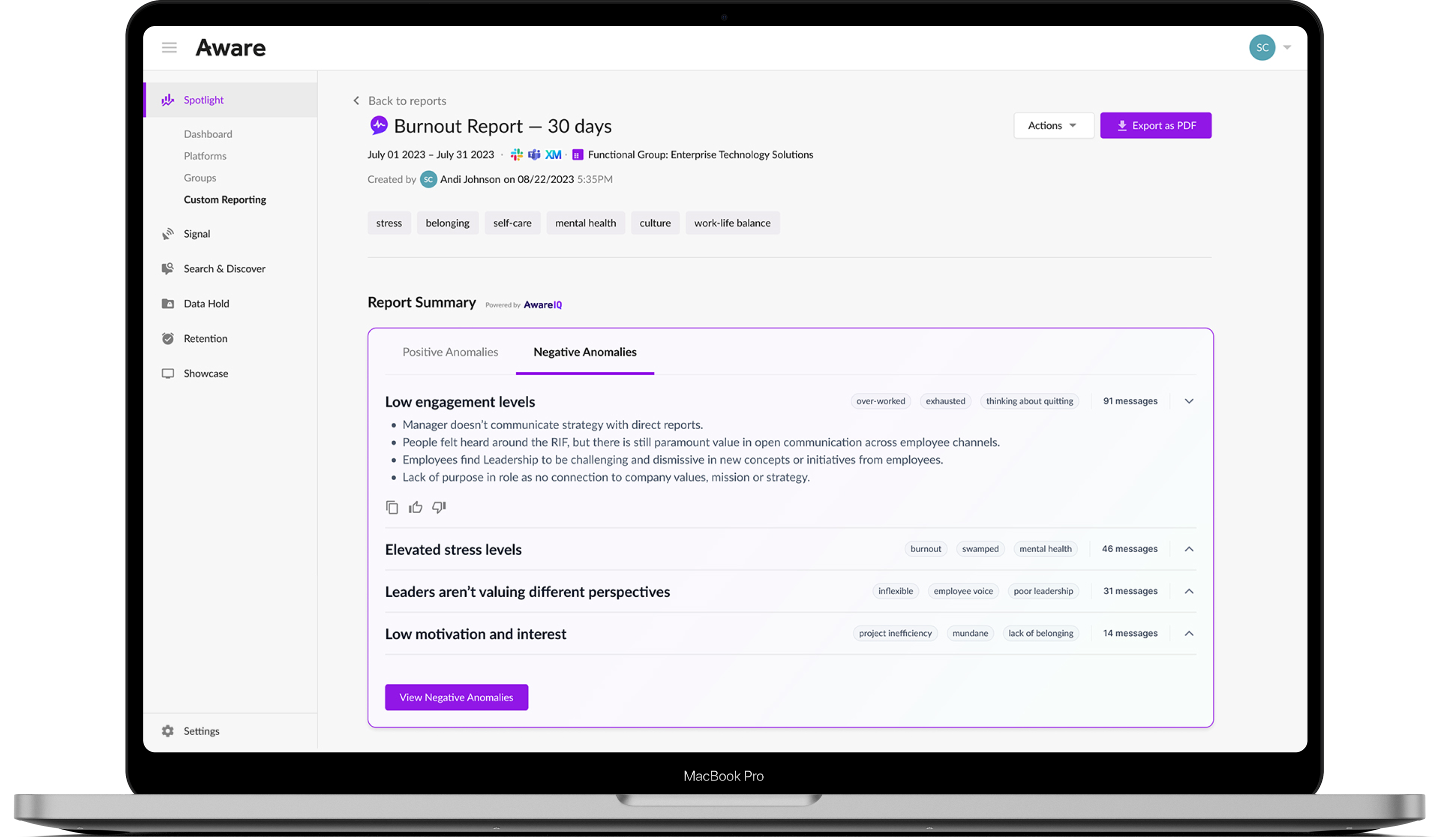
Introducing the industry's most accurate risk assessment calculator for digital workplaces
5. You need a plan to handle responsibility and bias
AI has received plenty of bad press for data scraping and privacy concerns, and business users must take these criticisms seriously before feeding their proprietary information into a machine. How does the AI handle and store your data? Does it use it for additional training and refinement? And can the end user control what data is and isn’t ingested?
In addition to these questions, AI buyers should also think about the unconscious biases that can surface in AI/ML models. Both Twitter (now X) and Zoom found themselves in hot water when their tech failed to identify Black faces in images, leading to only white people being shown in link previews or able to use virtual backgrounds during video calls.
Without careful consideration of potential blind spots, it’s easy to unintentionally train bias into an AI/ML model, which can then replicate and exacerbate real-world prejudices through its outputs.
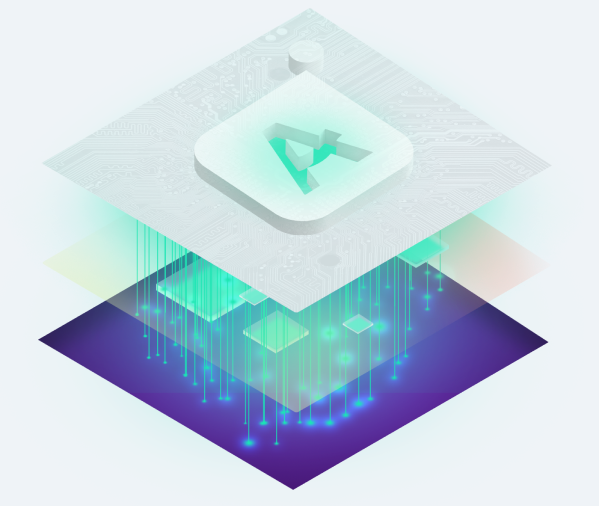
Learn more about Aware's industry-leading AI data platform
Final thoughts
Artificial intelligence has the power to revolutionize the way modern businesses run by harnessing the data created by the digital transformation. However, when considering making any AI/ML purchase for your business, it’s important to choose the right technology to suit your needs, goals, and budget, and ensuring that every new investment treats your sensitive and proprietary data with the respect it deserves.